Meta AI releases Web-SSL: A scalable and language-free method for learning visual representation

In recent years, contrasting language image models such as clipping have established themselves as the default choice for learning visual representations, especially in multimodal applications such as Visual Problems (VQA) and document comprehension. These models utilize large-scale image text pairs to incorporate semantic basis through language supervision. However, this dependence on text introduces conceptual and practical challenges: assuming that language is critical to multi-modal performance, obtaining the complexity of aligned datasets, and scalability limitations imposed by data availability. In contrast, visual self-supervised learning (SSL) (languageless operation) has historically demonstrated competitive outcomes in classification and segmentation tasks, but is granted multimodal inference due to performance gaps, especially in OCR and chart-based tasks.
Meta releases WebSSL model on hug surface (300m – 7b parameters)
To explore the ability of language-free visual learning on a large scale, Meta released Web-SSL Dino and Vision Transformer (VIT) model familyParameters ranging from 300 million to 7 billion are now available publicly by embracing faces. These models are trained only on a subset of images Metaclip Dataset (MC-2B)– A network-scale dataset containing 2 billion images. This controlled setting allows direct comparison between WebSSL and Clip, both trained with the same data, isolated the effect of language supervision.
The goal is not to replace the clip, but to strictly evaluate how far a pure visual self-division can go when the model and data scales are no longer limiting factors. This version represents an important step in understanding whether language supervision requires (or is merely beneficial) to train high-capacity vision encoders.
Technical architecture and training method
WebSSL includes two visual SSL paradigms: joint installation learning (via Dinov2) and mask modeling (via MAE). Each model followed a standardized training protocol using 224×224 resolution images and maintained a frozen vision encoder during downstream evaluation to ensure that the observed differences were attributed to preprocessing only.
The five capacity layers (VIT-1B to VIT-7B) were trained on the model using only unlabeled image data from MC-2B. use Cambrian-1a comprehensive 16-task VQA benchmark suite covering general vision understanding, knowledge-based reasoning, OCR and graph-based explanations.
Additionally, these models are locally supported in embracing faces transformers
Library that provides accessible checkpoints and seamlessly integrates into research workflows.
Performance insights and scaling behaviors
The experimental results reveal several key findings:
- Scaling model size: The WebSSL model demonstrates an almost logarithmic linear improvement in VQA performance as the parameter count increases. In contrast, the performance of the clip exceeds 3B parameters. WebSSL maintains competitive results across all VQA categories and shows significant growth in vision-centric and OCR and charting tasks on a larger scale.
- Data composition is important: WebSSS performs better than clips of OCR and chart tasks by filtering training data to contain only 1.3% text-rich images – +13.6% earnings from Ocrbench and ChartQA. This shows this Only the existence of visual textnot a language tag, can significantly improve task-specific performance.
- High resolution training: The WebSSL model is fine-tuned at a resolution of 518px, further narrowing the performance gap with high resolution models such as Siglip, especially high resolution models for document heavy tasks.
- LLM Alignment: Without any language supervision, WebSL shows increased consistency with validated language models (e.g. Llama-3) as model size and training exposure increases. This emerging behavior means that a larger vision model implicitly learns features related to good text semantics.
Importantly, WebSSL maintains strong performance on legacy benchmarks (Imagenet-1K classification, ADE20K breakdown, NYUV2 depth estimation) and generally outperforms MetaClip or even DINOV2 at equivalent settings.
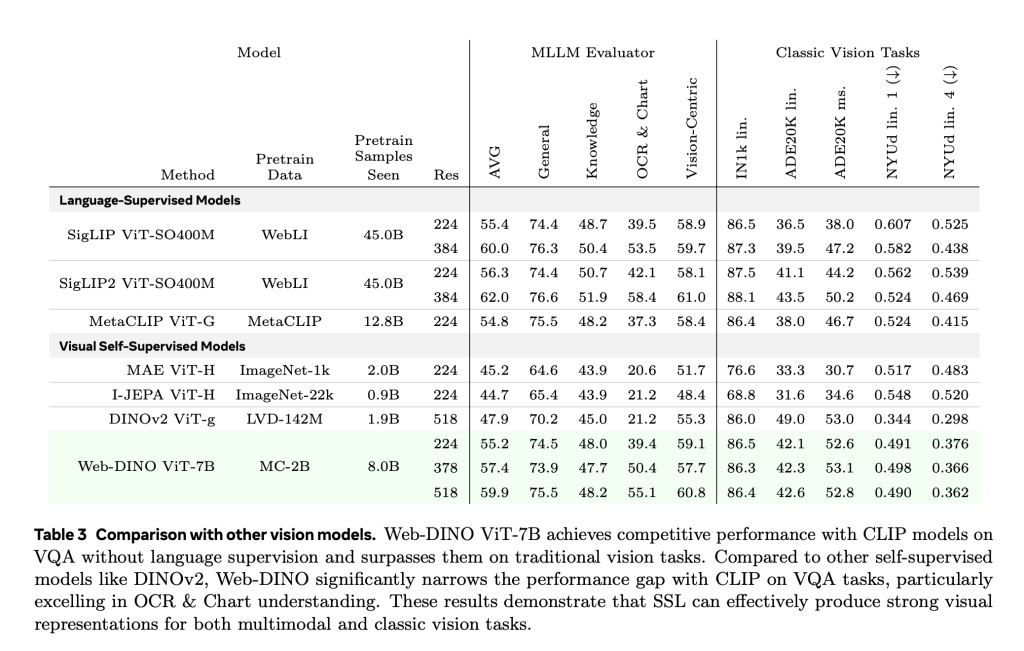
Summary observation
Meta’s Web-SSL study provides strong evidence Visual self-supervised learning appropriate scaling is a viable alternative to viable language preprocessing. These findings challenge the general assumption that language supervision is essential for multimodal understanding. Instead, they emphasize the importance of dataset composition, model scales, and careful evaluation across different benchmarks.
The release of models ranging from 300m to 7b parameters can enable broader research and downstream experiments without the limitations of paired data or proprietary pipelines. As the open source basis for future multimodal systems, the WebSSL model represents a meaningful advancement in scalable, language-free vision learning.
Check Model embracing face,,,,, Github page and Paper. Also, don’t forget to follow us twitter And join us Telegram Channel and LinkedIn GrOUP. Don’t forget to join us 90K+ ml reddit.
🔥 [Register Now] Minicon Agesic AI Virtual Conference: Free Registration + Certificate of Attendance + 4-hour Short Event (May 21, 9am-1pm) + Hands-On the Workshop
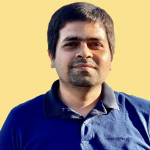
Asif Razzaq is CEO of Marktechpost Media Inc. As a visionary entrepreneur and engineer, ASIF is committed to harnessing the potential of artificial intelligence to achieve social benefits. His recent effort is to launch Marktechpost, an artificial intelligence media platform that has an in-depth coverage of machine learning and deep learning news that can sound both technically, both through technical voices and be understood by a wide audience. The platform has over 2 million views per month, demonstrating its popularity among its audience.
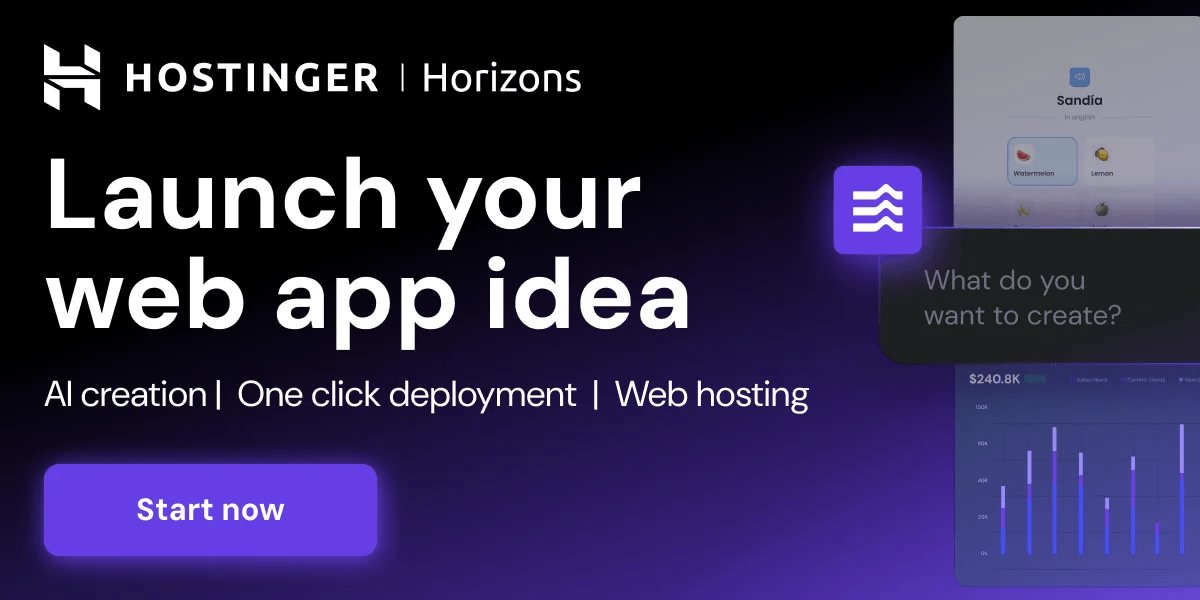