Skywork AI Advance Multimodal Inference: Introducing Skywork R1V2 through Hybrid Enhanced Learning

Latest advances in multimodal AI highlight an ongoing challenge: achieving strong professional reasoning capabilities while retaining generalizations across different tasks. “Slow Thinking” models such as OpenAI-O1 and Gemini-Inking have made progress in intentional analytical reasoning, but often exhibit impaired performance on general visual comprehension tasks and increase the tendency of visual hallucinations. As the field develops towards the establishment of general AI systems, reconciling this trade-off remains a key research question.
Skywork AI introduces Skywork R1V2
Skywork AI has released Skywork R1V2, a next-generation multi-modal inference model designed to systematically solve the trade-offs of inference generals. Based on the foundation of Skywork R1V, R1V2 introduces a hybrid enhanced learning framework that combines reward model guidance with signals based on structured rules. This model by learning directly from multimodal interactions, bypassing the traditional dependence on teacher distillation, thus providing open and repeatable progress by releasing the embracing face.
Technical methods and innovation
Skywork R1V2 combines group relative strategy optimization (GRPO) with selective sample buffer (SSB) to enhance training stability and efficiency. GRPO can perform relative evaluations between candidate responses in the same query group, but convergence problems can reduce effective learning signals. The SSB mechanism solves this problem by maintaining large numbers of information samples, thus ensuring continuous access to high-value gradients.
In addition, the model adopts a hybrid preference optimization (MPO) strategy that integrates reward model-based preferences with rule-based constraints. This hybrid optimization allows Skywork R1V2 to enhance step-by-step reasoning quality while maintaining consistency in general perceptual tasks. A modular training method that utilizes a lightweight adapter between the frozen intern VIT-6B vision encoder and the proven language model can maintain the inference capability of the language model while effectively optimizing cross-mode alignment.
Experience results and analysis
Skywork R1V2 shows strong performance in a range of inference and multi-mode benchmarks. On the text reasoning task, the model gets 78.9% on AIME2024, 63.6% on Livecodebench, 73.2% on LiveBench, 82.9% on IFEVAL and 66.3% on BFCL. These results represent a significant improvement in Skywork R1V1 and are competitive with a large number of larger models such as DeepSeek R1 (671B parameter).
In the multimodal evaluation, R1V2 accounted for 73.6% on MMMU, 74.0% on Mathvista, 62.6% on Olympic Banti there, 49.0% on MathVision, and 49.0% on MMMU-PRO accounted for 49.0%. This model is always superior to the open source baselines of comparable or larger sizes, including QWEN2.5-VL-72B and QVQ-PREVIEW-72B, which is especially excellent in tasks that require structured problems across visual and text input.
When compared with proprietary models, R1V2 shows a narrow performance gap. It surpasses the flash of Claude 3.5 sonnet and Gemini 2 on key multimodal benchmarks such as MMMU and Mathvista. Importantly, through the calibrated reinforcement strategy, the hallucination rate was significantly reduced to 8.7%, thus maintaining factual integrity as well as complex reasoning.
Qualitative evaluation further illustrates the systematic solution to R1V2, a model that demonstrates methodical decomposition and verification behaviors in complex scientific and mathematical tasks, thereby enhancing its consistency with reflective cognitive patterns.
in conclusion
Skywork R1V2 improves the state of multimodal reasoning with a well-designed hybrid enhanced learning framework. By addressing the problem of vanishing advantages of selective sample buffers and balancing the optimized signal through mixed preference optimization, the model has made significant improvements in both professional reasoning tasks and general multimodal understanding.
Skywork R1V2’s benchmark lead performance, such as 62.6% of the Olympiton Mountains and 73.6% of the MMMU establishes a strong open source baseline. Its design principles and training methods provide a pragmatic approach to developing powerful, effective multimodal AI systems. The future direction of Skywork AI includes enhancing general visual understanding while retaining the complex reasoning foundation laid by R1V2.
Check Paper and Embrace face model. Also, don’t forget to follow us twitter And join us Telegram Channel and LinkedIn GrOUP. Don’t forget to join us 90K+ ml reddit.
🔥 [Register Now] Minicon Agesic AI Virtual Conference: Free Registration + Certificate of Attendance + 4-hour Short Event (May 21, 9am-1pm) + Hands-On the Workshop
Sana Hassan, a consulting intern at Marktechpost and a dual-degree student at IIT Madras, is passionate about applying technology and AI to address real-world challenges. He is very interested in solving practical problems, and he brings a new perspective to the intersection of AI and real-life solutions.
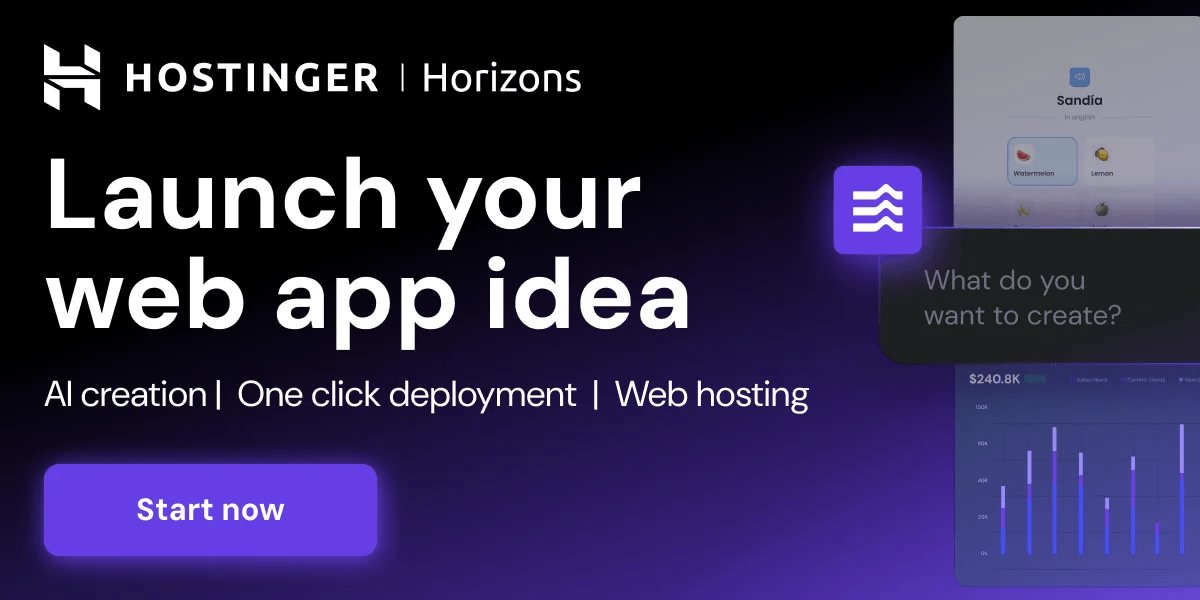