Microsoft AI releases PHI-4-ROUNTION: 14B parameter open weight inference model, achieving strong performance on complex inference tasks

Despite significant advances in large language models (LLMS), effective performance in inference-intensive tasks such as mathematical problem solving, algorithmic planning, or coding is limited by model size, training methods, and inference time functions. Models that perform well on general NLP benchmarks often lack the ability to construct multi-step reasoning chains or reflect on intermediate problem-solving states. Furthermore, while expanding the model size can improve inference capabilities, it introduces too good computing and deployment costs, especially for application in education, engineering and decision support systems.
Microsoft releases PHI-4 Inference Model Kit
Microsoft recently introduced the PHI-4 inference family, consisting of three models –PHI-4 Improvement,,,,, Phi-4-Reoning-plusand PHI-4-MINI-RENISING. These models are derived from the PHI-4 foundation (14B parameters) and are specially trained to handle complex reasoning tasks in mathematics, science and software-related problem solving. Each variant addresses different tradeoffs between computational efficiency and output accuracy. PHI-4 responses are optimized through supervised fine-tuning, while PHI-4-Reoning-Plus extends this with results-based reinforcement learning, especially for improved performance for high-variable tasks such as competitive-level mathematics.
The open weight model has been released, which contains transparent training details and evaluation logs, including benchmark designs, and is hosted in front of embrace, reproducibility and public access.
Technical composition and methodological advances
The PHI-4 response model is based on the PHI-4 architecture and has targeted improvements to model behavior and training systems. Key methodological decisions include:
- Structured Supervision Fine Tuning (SFT): More than 1.4 million prompts curated attention to the “boundary” case – the issue of the edge of the PHI-4 baseline function. Tips are purchased and filtered to emphasize multi-step reasoning rather than fact recall, and use O3-Mini synthetic responses in high voltage mode.
- After thinking about the chain format: To facilitate structured reasoning, the model was trained to use clear
Tags, encourage separation between the reasoning trajectory and the final answer. - Extended context processing: The rope base frequency has been modified to support a 32K token context window, allowing for a deeper solution trajectory, especially in multi-transform or elongated problem formats.
- Reinforcement Learning (PHI-4-Planning-Add): Using Group Relative Strategy Optimization (GRPO), PHI-4-ROSONING-PLUS further refined a set of approximately 6,400 math-centric problems. Bonus features were formulated to favor correct, concise and well-structured outputs while penalizing detailed, duplicate and format violations.
Data-centric and format-aware training systems support better time utilization and model generalization across domains, including invisible symbolic reasoning problems.
Evaluate and compare performance
Among the broad reasoning benchmarks, PHI-4-curatorial and PHI-4-ROOMISING-PLUS provide competitive results relative to significantly larger open weight models:
PHI-4-REONING-PLUS not only shows strong performance in the evaluation of specific areas, but also does not have clear training in these areas, but also performs well in program and combination issues such as TSP and 3SAT, such as TSP and 3SAT. Performance growth was also observed in the Teaching Follow-up (IFEVAL) and the Long Text QA (FLENQA), suggesting that thoughtful formulations improve the broader model utility.
Importantly, Microsoft reported full variance distributions in more than 50 generation runs for sensitive datasets such as AIME 2025, indicating that PHI-4-curated-matches or exceeds performance consistency for models like O3 Mini while still remaining disjointed with smaller baseline distributions such as DeepSeek-R1-distill.

Conclusion and meaning
The PHI-4 inference model represents a rigorous methodological effort to improve the capabilities of small models in structured reasoning. By combining data-centric training, architecture tuning, and minimal but bulls-eye good reinforcement learning, Microsoft demonstrates that 14B-scale models can match or outperform larger systems in tasks requiring multi-step reasoning and generalization.
The open weight availability and transparent benchmarking of the model sets precedent for future development of small LLMs, especially for application areas where interpretability, cost and reliability are critical. Future work is expected to extend reasoning capabilities to other STEM areas, improve decoding strategies, and explore scalable reinforcement learning over a longer period of time.
Check Paper, embrace the pages and Microsoft blogs. Also, don’t forget to follow us twitter And join us Telegram Channel and LinkedIn GrOUP. Don’t forget to join us 90K+ ml reddit.
🔥 [Register Now] Minicon Agesic AI Virtual Conference: Free Registration + Certificate of Attendance + 4-hour Short Event (May 21, 9am-1pm) + Hands-On the Workshop
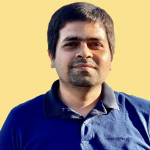
Asif Razzaq is CEO of Marktechpost Media Inc. As a visionary entrepreneur and engineer, ASIF is committed to harnessing the potential of artificial intelligence to achieve social benefits. His recent effort is to launch Marktechpost, an artificial intelligence media platform that has an in-depth coverage of machine learning and deep learning news that can sound both technically, both through technical voices and be understood by a wide audience. The platform has over 2 million views per month, demonstrating its popularity among its audience.
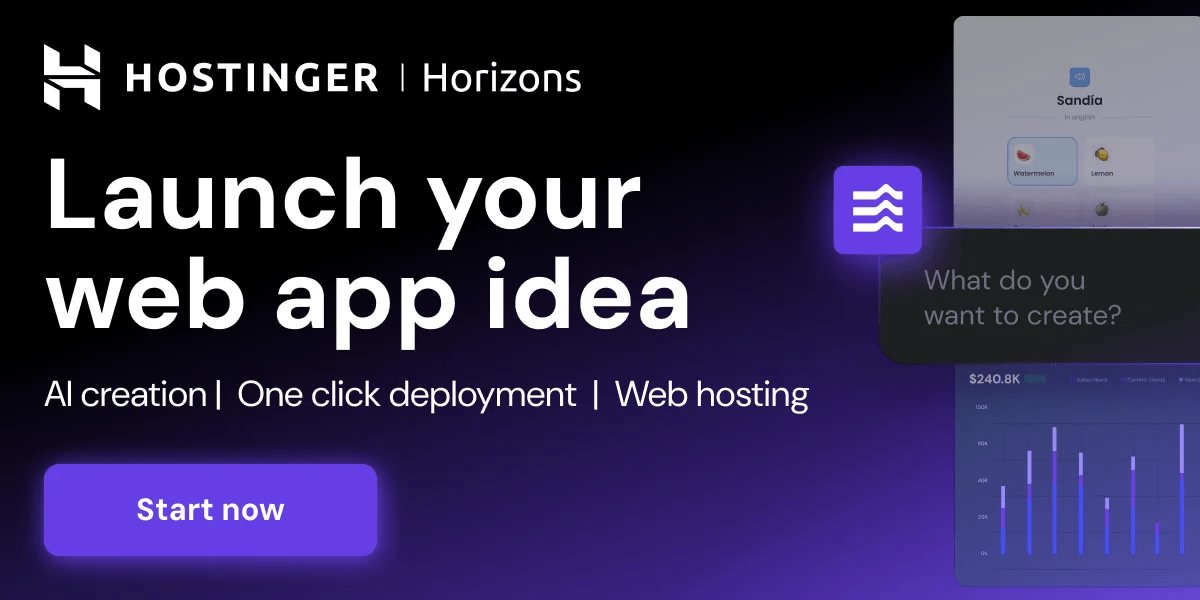