Multimodal LLM without compromise: Researchers from UCLA, UW-MADISON and ADOBE introduce X fusion to increase vision without losing language skills

LLM has made great progress in tasks such as language-related tasks such as dialogue AI, inference and code generation. However, human communication is beyond the scope of the text, often combining visual elements to enhance understanding. To create truly versatile AI, models need the ability to process and generate text and visual information simultaneously. This unified visual model is trained from scratch using autoregressive token prediction or hybrid approaches combined with methods such as diffusion and language loss. Nevertheless, it still requires a lot of computing resources and retrained for each new model. Another approach can adapt preprocessed LLMs to visual functions, which provides a more efficient path, but often compromises the original performance of the language model.
The current research focuses on three main strategies: merging LLM with independent image generation models, training large multimodal end-to-end, or combining diffusion and automatic regression losses. Although these methods have achieved latest results, they either require retraining large models or degrading the core functions of LLM. Despite these challenges, the increased visual components of LLM using validated LLM has shown great potential, especially in tasks involving image understanding and generation. However, these methods still face limitations in terms of efficiency and flexibility.
Researchers from UCLA, University of Wisconsin-Madison and Adobe Research have proposed X-fusion, which is suitable for pre-audited LLMSs to perform multimodal tasks while retaining language competence. X Fusion utilizes a dual architecture, freezes the LLM’s language weights while adding visually specific towers to process visual information. This approach aligns text and visual functions on multiple levels, improving the performance of image-to-text and text object tasks. Through ablation studies, the researchers highlighted the importance of clean image data for training and showed that combining visual features with pre-trained representations accelerates convergence, especially for smaller models.
X Fusion is a unified framework that adapts to audited LLMS for visual tasks while retaining its language capabilities. It uses a dual design design, freezing the text weight of the LLM while introducing a separate visual tower to process visual information. Use a pre-verified encoder to symbolize the image and jointly optimize the image and text tokens. The model combines optional X-Fuse operation to merge the capabilities of the two towers for enhanced performance. X-fusion is trained in self-cycle and image degradation losses, and its performance will be evaluated on image generation (text versus image) and image understanding (image versus text) tasks.
This study evaluates the twin tower architecture for alternative transformer variants targeting multimodal integration. It compares single tower, enclosed tower and dual projection designs, highlighting the flexibility of the double tower for image and text tasks. The double tower performed best in image generation and understanding, and performed better than 23% in FID without increasing training parameters. The study also examined the effects of noise and data comparison on performance and found that clean images can improve understanding and production. In addition, the visual functions are aligned with the outlined encoder and the like, such as clipping to improve performance, especially for smaller models.
In summary, X-fusion is a framework that adapts validated LLM to multimodal tasks such as image understanding and generation while preserving language capabilities. It introduces a two-tower architecture with language weights remaining fixed and provides a separate trainable visual tower to process visual features. Experimental results show that X fusion is superior to alternative designs in image and text object tasks. Key findings include merging to understand the data, the benefits of reducing noise in image data, and the positive impact of feature alignment, especially for smaller models. This study provides valuable insights into building effective multi-model models.
Check Paper. Also, don’t forget to follow us twitter.
Here is a brief overview of what we built in Marktechpost:
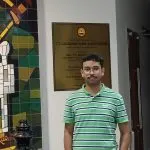
Sana Hassan, a consulting intern at Marktechpost and a dual-degree student at IIT Madras, is passionate about applying technology and AI to address real-world challenges. He is very interested in solving practical problems, and he brings a new perspective to the intersection of AI and real-life solutions.