Summary of Enhanced Language Model: Bridging the Disparity between Text Learning and Fine Tuning
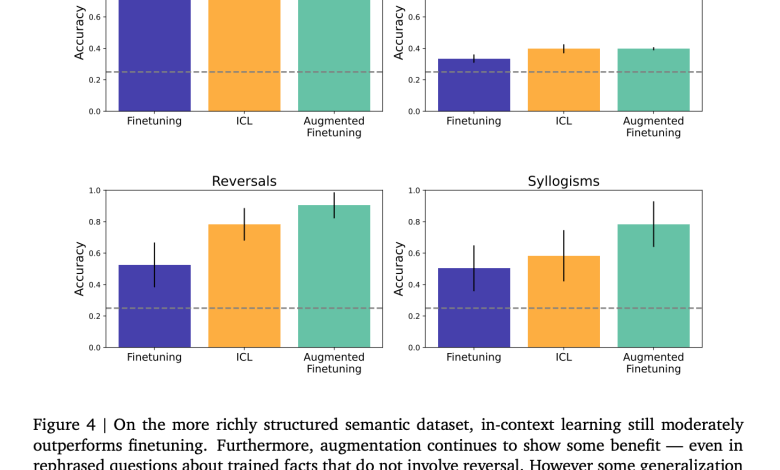
Language Models (LMS) have great functionality because they have excellent capabilities among text learners when estimating estimates in a huge Internet text corpus, allowing them to summarize effectively from only a few task examples. However, fine-tuning downstream tasks for these models presents significant challenges. While fine-tuning requires hundreds to thousands of examples, the resulting generalization pattern shows limitations. For example, the model fine-tunes statements like “B’s mother is A” to answer related questions such as “Who is A’s son?” However, LM can handle this inverse relationship in the context. This raises questions about the differences between text learning and fine-tuning generalization patterns and how these differences provide adaptive strategies for downstream tasks.
Research on improving LMS adaptability follows several key approaches. Cultural Learning Research studies learning and generalization patterns through empirical, mechanical and theoretical analysis. Mysterious learning research explores how to use information not explicitly included in the prompt. Data augmentation technology uses LLMS to improve the performance of finite data sets, while specific solutions target problems such as problems such as hard-coded enhancements, deductions, closed training, and generating inference pathways. In addition, synthetic data methods have evolved from early hand-designed data to improve generalizations in fields such as linguistics or mathematics to the latest methods of generating data directly from language models.
Researchers at Google DeepMind and Stanford University have built several datasets that isolate knowledge from preprocessed knowledge to create clean generalized tests. Performance of various generalization types is evaluated by exposing the trailer model to a subset of control information and by fine-tuning. Their findings suggest that intrinsic learning exhibits a more flexible generalization than fine-tuning in the data-matched settings, although in some exceptions, micro-tuning can generalize to reversals in larger knowledge structures. Building on these insights, the researchers developed a method to enhance the summary of fine-tuning by inferring in the fine-tuning data.
Researchers use well-designed multiple datasets to isolate specific generalization challenges or insert them into a wider learning environment. Evaluation relies on multiple choices’ probability scores without providing answer selection in context. The experiment involved a Gemini 1.5 flash using a batch size of 8 or 16 for fine-tuning. For text evaluation, researchers combined training documents as a context for guiding the moderation model and randomly sampled larger data sets by 8x to minimize interference problems. The key innovation is a dataset scaling approach that uses internal cultural generalizations to enhance fine-tune dataset coverage. This includes both local and global strategies, each with different environments and tips.
In the reversal curse dataset, close performance is achieved in reversal learning of reversals, while traditional fine-tuning shows near-zero accuracy as the model favors incorrect celebrity names seen during training. The enhanced data is fine-tuned by closed inference matching the high performance of pure intrinsic learning. Testing the reversal of simple nonsense reveals similar patterns, although the benefits are not obvious. For simple syllogisms, the validated model is executed at the opportunity level (indicating that there is no data pollution), but fine-tuning does produce a highly observed generalization of some syllogism types, while some logical inferences are consistent with simple language patterns. However, learning of intrinsic learning outperforms fine-tuning, and enhanced fine-tuning shows the best overall results.
In short, this article explores the generalized differences between cultural learning and fine-tuning when LMS faces a new information structure. The results show that, among some types of reasoning, excellent generalization of intrinsic learning has prompted researchers to develop methods that enhance fine-tuning performance by incorporating contextual inference into training data. Despite promising results, several limitations influenced the study. The first is a dependency on nonsense and incredible operations. Second, the focus of the study is on specific LM, limiting the generality of the results. Future research should examine learning and generalization differences between various models to extend these findings, especially new inference models.
View paper. All credits for this study are to the researchers on the project. Also, please feel free to follow us twitter And don’t forget to join us 95k+ ml reddit And subscribe Our newsletter.
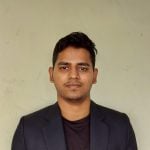
Sajjad Ansari is a final year undergraduate student from IIT Kharagpur. As a technology enthusiast, he delves into the practical application of AI, focusing on understanding AI technology and its real-world impact. He aims to express complex AI concepts in a clear and easy way.
🚨Build a Genai you can trust. ⭐️Parlant is your open source engine for controlled, compliance and purposeful AI conversations – Star Parlant on Github! (Promotion)