Bytedance releases UI-TARS-1.5: Open source multi-mode AI proxy builds on powerful visual models

Bytedance has released UI-TARS-1.5, an updated version of its multimodal proxy framework that focuses on graphical user interface (GUI) interactions and gaming environments. Designed as a visual model that perceives screen content and performs interactive tasks, UI-TARS-1.5 provides consistent improvements in a range of GUI automation and gaming inference benchmarks. It is worth noting that it surpasses several leading models, including the operator of OpenAI and human Claude 3.7, which can be accomplished in a variety of environments with accuracy and task completion.
This release continues to work on building a local proxy model, aiming to unify perception, cognition, and action through an integrated architecture that supports direct contact with GUI and visual content.
Local proxy method for GUI interaction
Unlike the tool-enhanced LLM or feature-name architecture, UI-TARS-1.5 is end-to-end trained, senses visual input (screenshot) and generates natively-like human-controlled actions such as mouse movement and keyboard input. This brings the model closer to how human users interact with digital systems.
UI-TARS-1.5 builds its predecessor by introducing several architectural and training enhancements:
- Perception and reasoning integration: The model co-codes screen images and text descriptions, supporting complex task understanding and visual grounding. Reasoning is supported by a multi-step “thinking” mechanism that distinguishes advanced planning from low-level execution.
- Unified action space: The operation representation is designed to be a platform that cannot be stationary and can have a consistent interface in desktop, mobile and gaming environments.
- Self-development through replaying traces: The training pipeline contains reflective online tracking data. This allows the model to iteratively refine its behavior by analyzing previous interactions, thereby reducing its dependence on planning demonstrations.
Together, these improvements enable UI-TARS-1.5 to support long horse interaction, error recovery and composition task planning – the most important UI navigation and control features.
Benchmarks and evaluations
The model has been evaluated on several benchmark suites that evaluate agent behavior in GUI and game-based tasks. These benchmarks provide a standard method for evaluating model performance in inference, grounding, and long-distance execution.
GUI Agent Tasks
- OSWorld (100 steps): The success rate of UI-TARS-1.5 was 42.5%, outperforming OpenAI operators (36.4%) and Claude 3.7 (28%). Benchmarks evaluate long article GUI tasks in synthetic OS environments.
- Windows Agent Arena (50 steps): The model scored 42.1%, significantly improving the previous baseline (e.g. 29.8%), demonstrating the powerful handling of desktop environments.
- Android World: The success rate of this model reached 64.2%, indicating the generality of mobile operating systems.
Visual grounding and screen understanding
- ScreenSpot-V2: The model achieves 94.2% accuracy in positioning GUI elements, over operators (87.9%) and Claude 3.7 (87.6%).
- ScreenSpotPro: In the more complex base benchmark, the UI-TARS-1.5 scored 61.6%, leading the operator (23.4%) and the Claude 3.7 (27.7%).

These results suggest consistent improvements in screen comprehension and action grounding, which is crucial for real-world GUI proxying.
Game environment
- Poki Games: UI-TARS-1.5 achieves 100% mission completion rate in 14 mini games. These games differ in mechanical and context, requiring models to span interactive dynamics.
- Minecraft (Minerl): This model achieved 42% success on mining tasks and 31% success on mob killing tasks when using the “Thingen-Act” module, suggesting that it can support advanced planning in an open environment.
Accessibility and tools
UI-TARS-1.5 is open sourced under the Apache 2.0 license and is available through multiple deployment options:
In addition to the model, the project provides detailed documentation, replay data and evaluation tools to facilitate experimentation and repeatability.
in conclusion
UI-TARS-1.5 is a technological advancement in the field of multi-mode AI agents, especially agents focusing on GUI control and grounded visual inference. Through a combination of visual integration, memory mechanisms and structured action planning, the model exhibits strong performance in a variety of interactive environments.
Rather than pursuing universality, the model is adapted to task-oriented multimodal reasoning, which can be realistic challenges of interacting with the software through visual understanding. Its open source version provides a practical framework for researchers and developers interested in exploring local proxy interfaces or automated interactive systems through language and vision.
Also, don’t forget to follow us twitter And join us Telegram Channel and LinkedIn GrOUP. Don’t forget to join us 90K+ ml reddit.
🔥 [Register Now] Minicon Agesic AI Virtual Conference: Free Registration + Certificate of Attendance + 4-hour Short Event (May 21, 9am-1pm) + Hands-On the Workshop
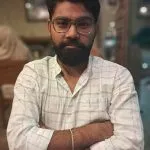
Nikhil is an intern consultant at Marktechpost. He is studying for a comprehensive material degree in integrated materials at the Haragpur Indian Technical College. Nikhil is an AI/ML enthusiast and has been studying applications in fields such as biomaterials and biomedical sciences. He has a strong background in materials science, and he is exploring new advancements and creating opportunities for contribution.
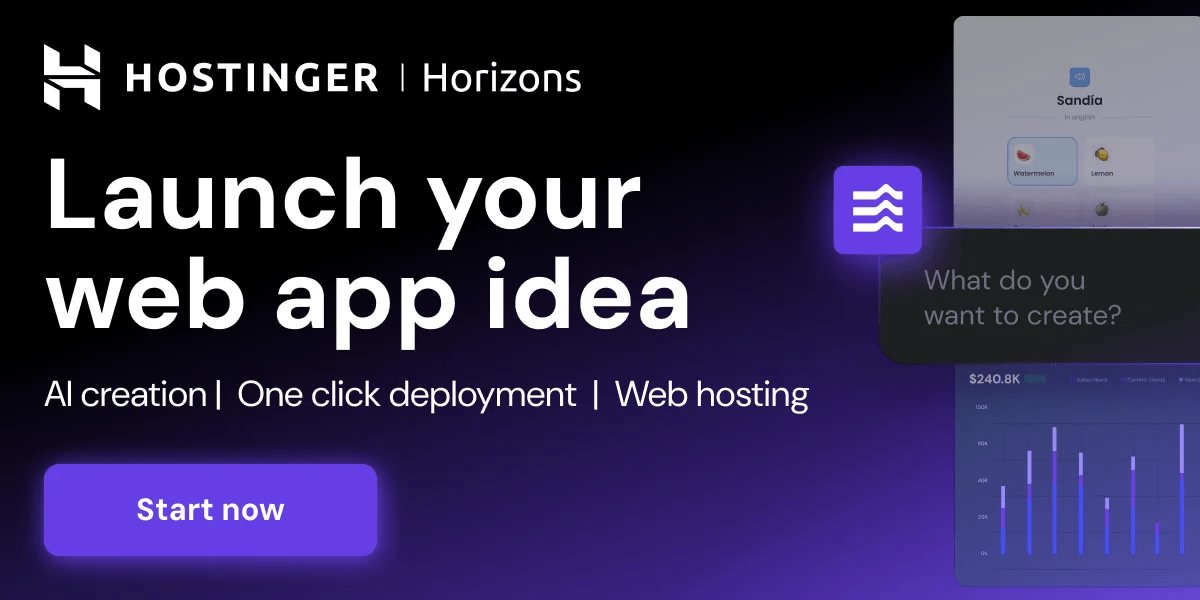