ATLA AI introduces ATLA MCP server: a local interface for a dedicated LLM judge through the Model Context Protocol (MCP)

Reliable evaluation of large language model (LLM) output is a critical but often complex aspect of AI system development. Integrating a consistent and objective evaluation pipeline into an existing workflow can introduce significant overhead. this ATLA MCP Server Solve this by exposing ATLA’s powerful LLM judge model (specifically targeting ratings and criticisms) Model Context Protocol (MCP). This locally compliant interface enables developers to seamlessly incorporate LLM evaluation into their tool and proxy workflows.
Model Context Protocol (MCP) as the basis
this Model Context Protocol (MCP) It is a structured interface that standardizes the interaction between LLM and external tools. By abstractly using tools in the use of tools behind the protocol, MCP disbands the logic of tool calls from the model implementation itself. The design promotes interoperability: any model capable of communicating with MCP can use any tool that reveals an MCP-compatible interface.
Based on this protocol, ATLA MCP server exposes evaluation capabilities in a consistent, transparent and easy to integrate into existing toolchains.
An overview of ATLA MCP server
ATLA MCP Server is a locally hosted service that provides direct access to evaluation models specifically used to evaluate LLM output. It is compatible with a range of development environments and supports integration with the following tools:
- Claude Desktop: Enable evaluation in a conversation environment.
- cursor: Allows edit ratings of code segments based on specified criteria.
- Openai Agent SDK: Promote programmatic assessment before decision-making or output dispatch.
By integrating the server into existing workflows, developers can use reproducible and version-controlled processes to perform structured evaluation of model output.
Special evaluation model
The core of the ATLA MCP server includes two dedicated evaluation models:
- Selene 1: A well-trained full-capacity model for evaluation and criticism tasks.
- Selene Mini: A resource efficiency variant designed to reason faster with reliable scoring capabilities.
Which Selene model does an agent use?
If you do not want to leave model selection to the proxy, you can specify the model.
Unlike the general LLM evaluated by cue inference simulations, the Selene model is optimized to produce consistent, low-change assessments and detailed criticisms. This reduces reinforcement such as self-consistent bias or incorrect reasoning.
Evaluation APIs and tools
The server exposes two major MCP compatibility evaluation tools:
- estuatue_llm_response: Score a single model response based on user-defined criteria.
- estuatue_llm_response_on_multiple_criteria: Enable multidimensional evaluation by scoring on several independent criteria.
These tools support fine-grained feedback loops that can be used to implement self-correction behavior in a proxy system or to verify output before user exposure.
Demo: Feedback Loop in Practice
use Claude Desktop Connect to the MCP server, we require that the model be Pokémon Charizard. Then evaluate using the generated name Selene Meet two criteria: originality and humor. According to criticism, Claude modified the name accordingly. This simple loop shows how the agent dynamically improves the output using structured automatic feedback (no manual intervention required).
While this is an example of intentional playfulness, the same evaluation mechanism applies to more practical use cases. For example:
- exist Customer SupportAgents can self-evaluate their responses before submission, which helps to harmonize policies.
- exist Code Generation Workflowthe tool can score the generated summary for correctness, security, or style compliance.
- exist Enterprise content generationTeams can automate inspections to ensure clarity, factual accuracy and brand consistency.
These schemes demonstrate the broader value of integrating ATLA’s evaluation model into production systems, allowing for good quality assurance between different LLM-driven applications.
Settings and configuration
Get started with the ATLA MCP server:
- Get API keys from the ATLA dashboard.
- Clone the GITHUB repository and follow the “Installation Guide”.
- Connect your MCP compatible client (Claude, Cursor, etc.) to start issuing evaluation requests.
The server is built to support direct integration into the proxy runtime and IDE workflow with minimal overhead.
Development and future direction
The ATLA MCP server was developed in collaboration with AI systems such as Claude to ensure compatibility and functional strength in real-world applications. This iterative design approach allows for effective testing of evaluation tools in the same environment they intend to use.
Future enhancements will focus on expanding the scope of support evaluation types and improving interoperability through other customer and orchestration tools.
To contribute or provide feedback, visit the ATLA MCP server GitHub. Developers are encouraged to try servers, report issues and explore use cases in the broader MCP ecosystem.
notes: Thanks to the ATLA AI team for this article’s thought leadership/resources. The Atla AI team supports us in providing this content/article.
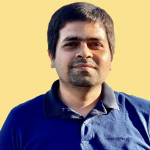
Asif Razzaq is CEO of Marktechpost Media Inc. As a visionary entrepreneur and engineer, ASIF is committed to harnessing the potential of artificial intelligence to achieve social benefits. His recent effort is to launch Marktechpost, an artificial intelligence media platform that has an in-depth coverage of machine learning and deep learning news that can sound both technically, both through technical voices and be understood by a wide audience. The platform has over 2 million views per month, demonstrating its popularity among its audience.
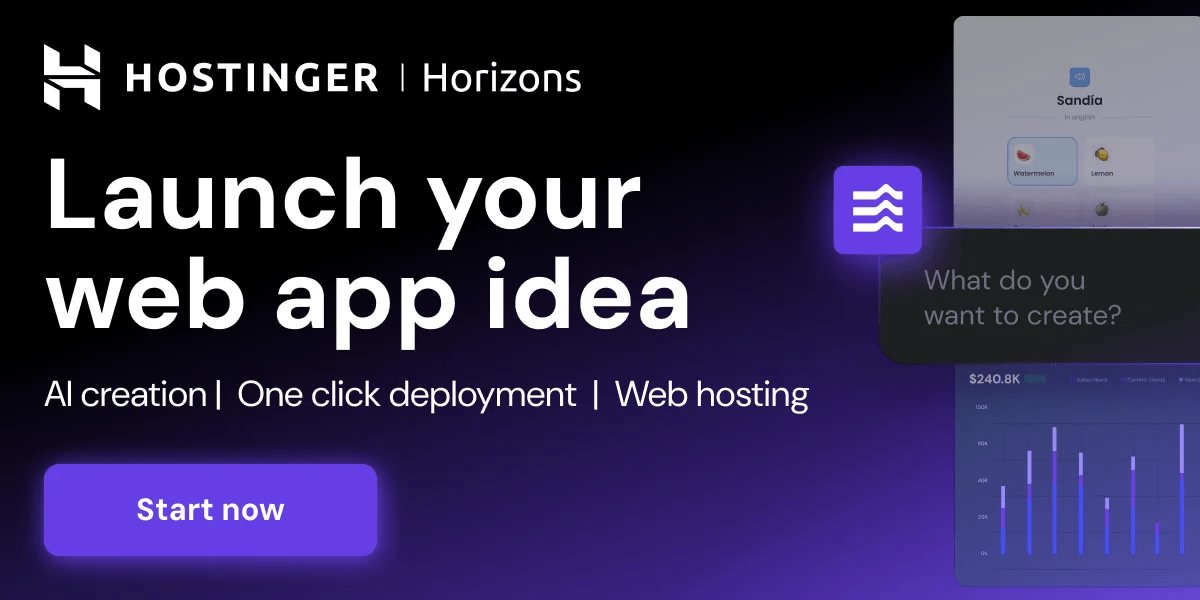