Can coding agents improve themselves? Researchers at the University of Bristol and IGENT AI have proposed SICA (self-improved coding agent) to iteratively enhance their own code and performance

Development of agent systems – LLM embedded in scaffolding that enables tools and autonomous decision making, significant progress has been made. However, most implementations today rely on fixed manual orchestration strategies. These designs are inherently limited, limiting the agency’s adaptability to new tasks and environments. As the model grows in function, the rigidity of its execution framework becomes a bottleneck, especially in areas such as software engineering, where task complexity and variability require more flexible systems.
In response, researchers from the University of Bristol and IGENT AI presented Sika (Self-improved Coding Proxy) – A novel proxy architecture designed to iteratively enhance its own performance by modifying its underlying code. Unlike previous methods (such as ADA), are the responsibilities between the meta-agent and the target agent, and SICA unifies these roles. The same agent performing the task is also responsible for evaluating past performance, identifying shortcomings and updating their own implementations. This integration allows for a continuous cycle of self-guided without external intervention.
Self-improved buildings and mechanisms
SICA is built on a minimal, scalable base proxy, equipped with tools to manipulate its code base, navigate directories, execute shell commands, and call child proxy. Its architecture follows a loop: evaluation, selection, modification. In each iteration, the agent performs on predefined tasks based on their own performance, stores the results, and selects the most efficient prior version as the basis for further improvement.
The agent uses utility functions to evaluate performance, which combines accuracy, time and cost metrics. Key components include:
- Sub-agent structure Used to decompose problems and manage context in LLM constraints.
- Asynchronous supervisiona monitoring LLM thread to ensure that the agent stops execution and stops execution in non-conducting or diverging situations.
- Self-editing functionwith the help of SmartEditor, AST-based symbol locator and DIFF digest, the proxy behavior can be accurately modified.
This structure allows agents to perform controlled experiments on their own designs and deploy updates to improve results.

Experience Assessment
The researchers evaluated several code-related benchmarks for SICA, including a subset of the proven SWE benchmarks, LiveCodeBench, and synthesis tasks focused on file editing and symbol position. The results show measurable benefits in the iteration. For example, the accuracy of the verified SWE benchmark increased from 17% to 53% and file editing performance increased from 82% to 94%.
These improvements are not limited to benchmark scores. Agents also optimize execution latency and resource efficiency, reducing the average cost and time per task. It is worth noting that improvements are not the result of the basic LLM weight update, but are achieved by changing tool orchestration, file management strategies, and problem-decomposition heuristics.
However, the benefits on reasoning-dominated tasks such as AIME and GPQA are less obvious. In these cases, the performance of the base LLM (such as O3-Mini) is already close to the task cap, thus limiting the marginal benefits of other scaffolding. Furthermore, the introduction of certain tool-based inference steps seems to undermine the performance of the validated inference model, suggesting the need for more integrated co-training between proxy logic and model behavior.
in conclusion
The SICA framework illustrates the specific path to autonomous improvement of the proxy system. By consolidating execution and self-editing in a single agent, the system avoids many of the pitfalls of manual design and implements empirical feedback-driven iterative refinement. The results show that this approach is feasible, especially in the domain with long horse, tool-mediated tasks (e.g., software engineering).
Although there are clear boundaries in the effectiveness of scaffolding improvements alone (especially for tasks dominated by pure reasoning), this study lays the foundation for future work on hybrid optimization, with both model and agent design developing together. SICA also uses LLM-based supervisors and structured execution traces to ensure transparency and control, thus introducing practical considerations for security and observability in self-improvement systems.
Check Paper and github pages. Also, don’t forget to follow us twitter And join us Telegram Channel and LinkedIn GrOUP. Don’t forget to join us 90K+ ml reddit.
🔥 [Register Now] Minicon Agesic AI Virtual Conference: Free Registration + Certificate of Attendance + 4-hour Short Event (May 21, 9am-1pm) + Hands-On the Workshop
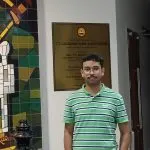
Sana Hassan, a consulting intern at Marktechpost and a dual-degree student at IIT Madras, is passionate about applying technology and AI to address real-world challenges. He is very interested in solving practical problems, and he brings a new perspective to the intersection of AI and real-life solutions.
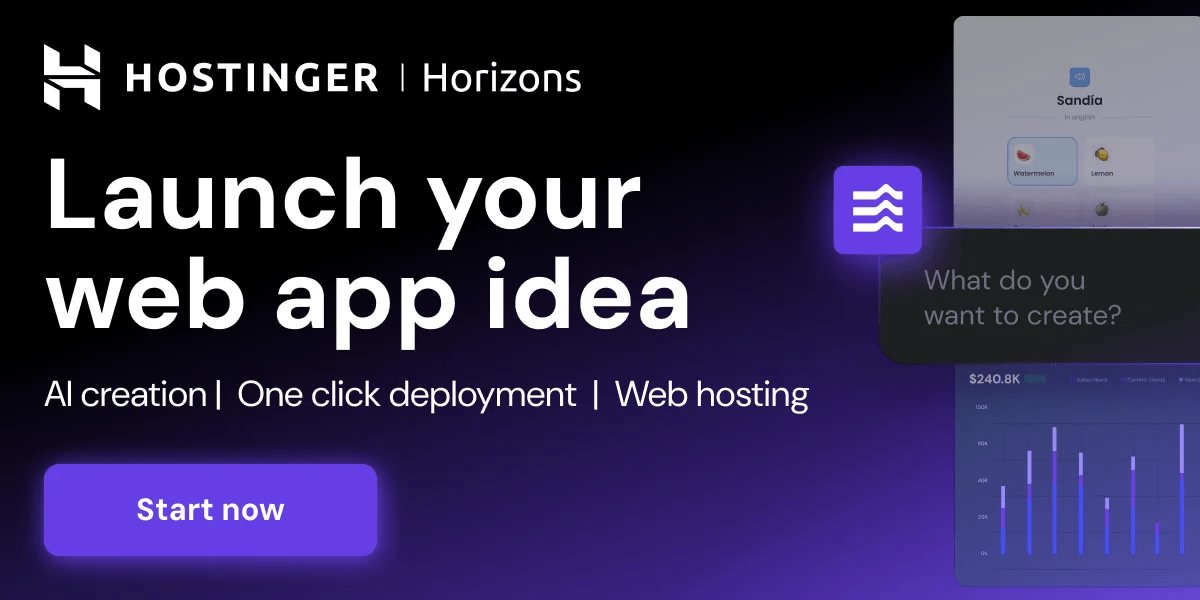