How to create a custom model context protocol (MCP) client using Gemini

In this tutorial, we will implement a custom model context protocol (MCP) client using Gemini. By the end of this tutorial, you will be able to connect your AI applications to your MCP server, unzipping powerful new features to enhance your project.
Gemini API
We will use the Gemini 2.0 Flash model in this tutorial.
To get the Gemini API key, visit Google’s Gemini API Key page and follow the instructions.
Once you have the key, store it safely – you need it later.
node.js
Some MCP servers require Node.js to run. Download the latest news Version nodejs.org’s node.js
- Run the installer.
- Take all settings as default settings and complete the installation.
National Park Services API
For this tutorial, we will expose the National Park Services MCP server to our customers. To use the National Park Services API, you can request the API key by visiting this link and filling in a short form. Once submitted, the API key will be sent to your email.
Make sure to make this key accessible – we will use it soon.
Install Python library
In the command prompt, enter the following code to install the Python library:
pip install mcp python-dotenv google-genai
Create an MCP.JSON file
Next, create a file named MCP.JSON.
This file will store configuration details about the MCP server to which the client will connect.
After creating the file, add the following initial content:
{
"mcpServers": {
"nationalparks": {
"command": "npx",
"args": ["-y", "mcp-server-nationalparks"],
"env": {
"NPS_API_KEY":
}
}
}
}
replace
Create .ENV file
Create a .ENV file in the same directory as the MCP.JSON file and enter the following code:
replace
Now we will create a Client File to implement our MCP client. Make sure this file is MCP.JSON and .env
Basic customer structure
We will first import the necessary libraries and create a basic client class
import asyncio
import json
import os
from typing import List, Optional
from contextlib import AsyncExitStack
import warnings
from google import genai
from google.genai import types
from mcp import ClientSession, StdioServerParameters
from mcp.client.stdio import stdio_client
from dotenv import load_dotenv
load_dotenv()
warnings.filterwarnings("ignore", category=ResourceWarning)
def clean_schema(schema): # Cleans the schema by keeping only allowed keys
allowed_keys = {"type", "properties", "required", "description", "title", "default", "enum"}
return {k: v for k, v in schema.items() if k in allowed_keys}
class MCPGeminiAgent:
def __init__(self):
self.session: Optional[ClientSession] = None
self.exit_stack = AsyncExitStack()
self.genai_client = genai.Client(api_key=os.getenv("GEMINI_API_KEY"))
self.model = "gemini-2.0-flash"
self.tools = None
self.server_params = None
self.server_name = None
The __init__ method initializes McPgeminiagent by setting up an asynchronous session manager, loading the Gemini API client and preparing model configuration, tool and server details for placeholders.
It lays the foundation for managing server connections and interacting with the Gemini model.
Select MCP Server
async def select_server(self):
with open('mcp.json', 'r') as f:
mcp_config = json.load(f)
servers = mcp_config['mcpServers']
server_names = list(servers.keys())
print("Available MCP servers:")
for idx, name in enumerate(server_names):
print(f" {idx+1}. {name}")
while True:
try:
choice = int(input(f"Please select a server by number [1-{len(server_names)}]: "))
if 1
This method prompts the user to select a server from the available options listed in MCP.JSON. It loads and prepares the connection parameters of the selected server for later use.
Connect to the MCP server
async def connect(self):
await self.select_server()
self.stdio_transport = await self.exit_stack.enter_async_context(stdio_client(self.server_params))
self.stdio, self.write = self.stdio_transport
self.session = await self.exit_stack.enter_async_context(ClientSession(self.stdio, self.write))
await self.session.initialize()
print(f"Successfully connected to: {self.server_name}")
# List available tools for this server
mcp_tools = await self.session.list_tools()
print("nAvailable MCP tools for this server:")
for tool in mcp_tools.tools:
print(f"- {tool.name}: {tool.description}")
This can use STDIO transport to establish an asynchronous connection to the selected MCP server. It initializes the MCP session and retrieves available tools from the server.
Handle user queries and tool calls
async def agent_loop(self, prompt: str) -> str:
contents = [types.Content(role="user", parts=[types.Part(text=prompt)])]
mcp_tools = await self.session.list_tools()
tools = types.Tool(function_declarations=[
{
"name": tool.name,
"description": tool.description,
"parameters": clean_schema(getattr(tool, "inputSchema", {}))
}
for tool in mcp_tools.tools
])
self.tools = tools
response = await self.genai_client.aio.models.generate_content(
model=self.model,
contents=contents,
config=types.GenerateContentConfig(
temperature=0,
tools=[tools],
),
)
contents.append(response.candidates[0].content)
turn_count = 0
max_tool_turns = 5
while response.function_calls and turn_count = max_tool_turns and response.function_calls:
print(f"Stopped after {max_tool_turns} tool calls to avoid infinite loops.")
print("All tool calls complete. Displaying Gemini's final response.")
return response
This method sends user prompts to Gemini, processes any tool calls returned by the model, executes the corresponding MCP tool, and iteratively improves the response. It manages multi-turn interactions between Gemini and server tools.
Interactive chat loop
async def chat(self):
print(f"nMCP-Gemini Assistant is ready and connected to: {self.server_name}")
print("Enter your question below, or type 'quit' to exit.")
while True:
try:
query = input("nYour query: ").strip()
if query.lower() == 'quit':
print("Session ended. Goodbye!")
break
print(f"Processing your request...")
res = await self.agent_loop(query)
print("nGemini's answer:")
print(res.text)
except KeyboardInterrupt:
print("nSession interrupted. Goodbye!")
break
except Exception as e:
print(f"nAn error occurred: {str(e)}")
This provides a command line interface where users can continually submit queries from Gemini and receive answers until they exit the session.
Clean up resources
async def cleanup(self):
await self.exit_stack.aclose()
This closes the asynchronous context and cleans up all open resources such as sessions and connections stack gracefully.
Main entrance points
async def main():
agent = MCPGeminiAgent()
try:
await agent.connect()
await agent.chat()
finally:
await agent.cleanup()
if __name__ == "__main__":
import sys
import os
try:
asyncio.run(main())
except KeyboardInterrupt:
print("Session interrupted. Goodbye!")
finally:
sys.stderr = open(os.devnull, "w")
This is the main execution logic.
Except main(), all other methods are McPgeminiagent class. You can find the full client.py file here.
Run the following prompts in the terminal to run your client:
The client will:
- Read the MCP.JSON file to list the different available MCP servers.
- Prompt the user to select one of the listed servers.
- Connect to the selected MCP server using the provided configuration and environment settings.
- Interact with the Gemini model through a series of queries and responses.
- Allows users to publish prompts, execute tools, and process responses using the model.
- Provides a command line interface for users to participate in the system and receive real-time results.
- After the session is over, make sure the resources are cleaned correctly, the connection is closed and the memory is freed.

I am a civil engineering graduate in Islamic Islam in Jamia Milia New Delhi (2022) and I am very interested in data science, especially neural networks and their applications in various fields.
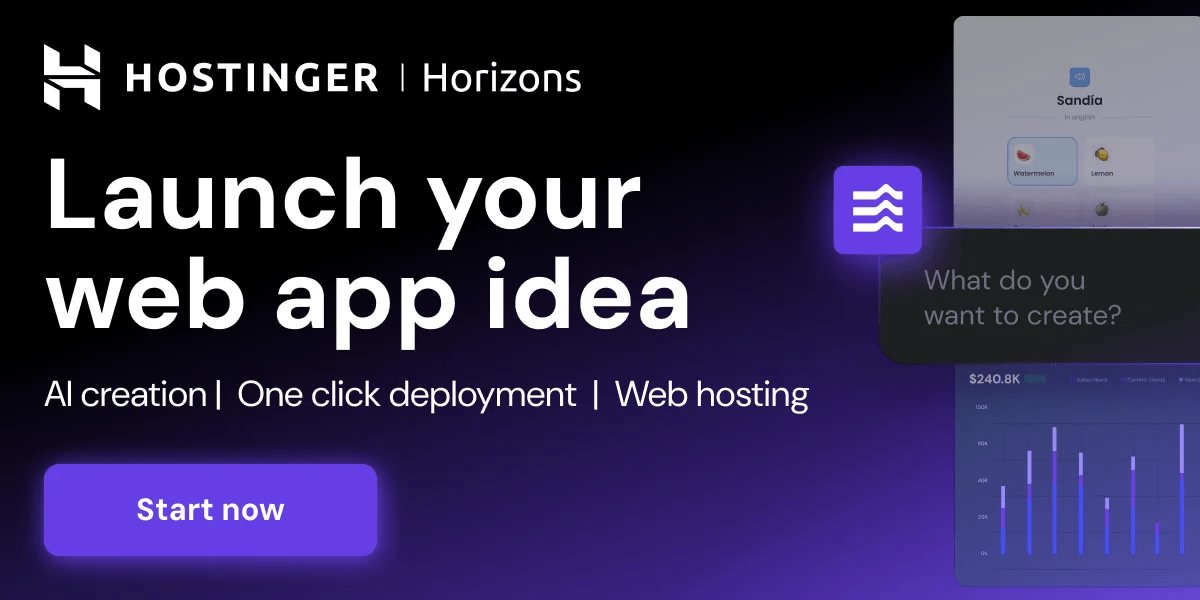