Multimodal query requires multimodal rags: Researchers from Kaist and Deepauto.ai proposed Universalrag, a new framework that dynamically spans modality and particle size to accurately and efficiently retrieve power generation

It turns out that by receiving its output to external, relevant information, the factual accuracy of LLM can be effectively improved. However, most existing RAG implementations are limited to text-based corpus, which limits its applicability to the realities where the query may require various information, from text definitions to spatial understanding from images or time reasoning in videos. Although some recent methods have extended rags to handle different modes such as images and videos, these systems are often limited to running in a single modal specific corpus. This limits their ability to effectively respond to various user queries that require multimodal reasoning. Furthermore, current rag methods are often retrieved from various ways without discrimination, which is most relevant to a given query, thus making the process inefficient and less adaptable to specific information needs.
To address this problem, recent research highlights the need for adaptive rag systems to determine appropriate methods and retrieval granularity based on the query environment. Strategies include complexity-based routing queries, such as deciding between no retrieval, single-step or multi-step retrieval and using model confidence to trigger retrieval only if needed. Furthermore, the granularity of the search plays a crucial role, as studies have shown that indexing corpus on higher quality levels (such as propositions or specific video clips) can significantly improve retrieval relevance and system performance. Therefore, in order to truly support complex real-life information needs, it must handle multiple ways and adjust its retrieval depth and scope to the specific needs of each query.
Researchers at Kaist and Deepauto.ai introduced Universalrag, a rag frame that retrieves and integrates knowledge from various modal-specific sources (text, images, videos) and multiple granularity levels. Unlike traditional methods, embedding all patterns into shared spaces, resulting in modal bias, Universalrag uses a modal-aware routing mechanism to dynamically select the most relevant corpus based on query. It further improves retrieval accuracy by organizing each method into a granular corpus, such as paragraphs or video clips. Universalrag consistently outperforms unified and modal-specific benchmarks in eight multimodal benchmarks, demonstrating its adaptability to various query requirements.
Universalrag is a search-based generation framework that can handle queries in various ways and data granularity. Unlike the standard rag model limited to a single corpus, Universal separates knowledge into text, image and video corpus, each with fine-grained and coarse grades. The routing module first determines the best way and granularity for a given query, selects between options such as paragraphs, complete documents, video clips, or full videos, and retrieves relevant information accordingly. The router can be an LLM-free classifier or a trained model using heuristic labels in the benchmark dataset. The LVLM then generates the final response using the selected content.
The experimental setup evaluated Universal Studios in six search scenarios: no searches, paragraphs, documents, images, clips, and videos. For none return, MMLU test common sense. Paragraph-level tasks use squads and natural problems, while HotPotQA handles retrieval of multi-hop documents. Image-based queries are from WebQA, and video-related queries are from LVBench and Videorag datasets, divided into editing and full video levels. The corresponding search corpus is curated for each mode – YouTube videos based on Wikipedia’s text, image WebQA and video tasks. This comprehensive benchmark ensures a powerful assessment of various methods and retrieval granularity.
In short, Universalrag is a search-based generation framework that can retrieve knowledge from a variety of ways and granularity levels. Unlike existing rag methods that rely on a single (usually text-only) corpus or single-mode source, Universal routes the query’s queries dynamically to the most appropriate modal and granularity-specific corpus. This approach solves problems such as modal gaps and strict retrieval structures. In eight multimodal benchmarks, Universalrag was evaluated on eight multimodal benchmarks, outperforming unified and modal specific benchmarks. The study also highlights the benefits of fine-grained retrieval and highlights how well-trained and train-free routing mechanisms contribute to robust, flexible multimodal reasoning.
Check Paper. Also, don’t forget to follow us twitter And join us Telegram Channel and LinkedIn GrOUP. Don’t forget to join us 90K+ ml reddit. To promote and partnership, Please talk to us.
🔥 [Register Now] Minicon Agesic AI Virtual Conference: Free Registration + Certificate of Attendance + 4-hour Short Event (May 21, 9am-1pm) + Hands-On the Workshop
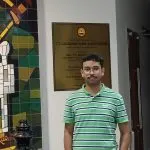
Sana Hassan, a consulting intern at Marktechpost and a dual-degree student at IIT Madras, is passionate about applying technology and AI to address real-world challenges. He is very interested in solving practical problems, and he brings a new perspective to the intersection of AI and real-life solutions.