NVIDIA AI introduces Audio-SD: A unified diffusion-based framework for rapidly guiding audio synthesis and source separation without the need for specialized data sets

Audio diffusion models have achieved high-quality synthesis of speech, music and Foley sounds, but they are mainly excellent in sample generation rather than parameter optimization. Tasks such as physically informed influence sound generation or timely driven source separation require models to adjust explicit, interpretable parameters under structural constraints. Score Distillation Sampling (SDS) (SDS) (backpropagation through preprocessing diffusion priors, it has powered text to 3D and image editing), but has not been applied to audio yet. Adapting SDS to audio diffusion allows optimization of parameter audio representations without the need to assemble large task-specific datasets, bridging modern generative models with parameterized synthesis workflows.
Classic audio technology (such as frequency modulation (FM) synthesis) uses an operator-modulated oscillator to create rich tones and perform physically grounded impact simulators – providing a compact, interpretable parameter space. Likewise, source separation has evolved from matrix decomposition to neural and text-guided methods for isolating components such as vocals or instruments. By integrating SDS updates with preprocessed audio diffusion models, the generative priors of learning can be leveraged to guide FM parameters, impact sound simulators, or optimization of separation masks directly from advanced cues, combining interpretive unity of signal processing with flexibility based on modern diffusion generation.
Researchers at NVIDIA and MIT introduced Audio-SD, an SDS extension for text-conditioned audio diffusion models. Audio-SD uses a single validated model to perform various audio tasks without the need for a dedicated data set. The generation priors are refined into parameter audio representations, facilitating tasks such as impact sound simulation, FM synthesis parameter calibration, and source separation. The framework combines data-driven priors with clear parameter control to produce compelling results. Major improvements include stable decoder-based SD, multi-step degradation, and multi-scale spectrogram approaches to provide better high-frequency details and realism.
This study discusses the application of SD to audio diffusion models. Inspired by DreamFusion, SDS generates volume audio through rendering functions, improves performance by bypassing the encoder gradient, and instead focuses on decoded audio. The method is enhanced by three modifications: avoiding the instability of the encoder, emphasizing the spectrogram to highlight high-frequency details, and using multi-step degradation for improved stability. Audio-SD applications include FM synthesizers, impact sound synthesis and source separation. These tasks show how SD adapts to different audio domains without retraining, ensuring that the synthesized audio is consistent with the text cues while maintaining high loyalty.
The performance of the audio-SD framework was demonstrated in three tasks: FM synthesis, impact synthesis and source separation. The experiment aims to test the effectiveness of the framework using subjective (hearing test) and objective indicators such as clapping scores, distance to ground truth and signal-to-noise ratio (SDR). Verified models (such as stable audio on checkpoints) are used for these tasks. The results show significant improvements in audio synthesis and separation and are clear with text prompts.
In summary, this study introduces audio-SD, an approach that extends SD to an audio diffusion model of text conditions. Audio SD can implement various tasks using a single predicted model, such as simulating physically informed influence sound, adjusting FM synthesis parameters, and prompt-based source separation. This method unifies data-driven priors using user-defined representations, eliminating the need for large domain-specific datasets. Despite challenges in model coverage, potential coding artifacts, and optimization sensitivity, Audio SD demonstrates the potential of multimodal research based on distillation, especially in audio-related tasks.
Check Paper and project pages. All credits for this study are to the researchers on the project. Also, please feel free to follow us twitter And don’t forget to join us 90K+ ml reddit.
Here is a brief overview of what we built in Marktechpost:
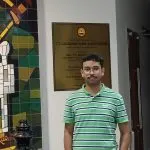
Sana Hassan, a consulting intern at Marktechpost and a dual-degree student at IIT Madras, is passionate about applying technology and AI to address real-world challenges. He is very interested in solving practical problems, and he brings a new perspective to the intersection of AI and real-life solutions.