Reinforcement Learning for Email Agents: The Art of OpenPipe• Precision, Delay and Cost Better than O3

OpenPipe launches Art·e (an automated search tool for email), an open source research agent designed to answer user questions based on inbox content, with a focus on accuracy, responsiveness, and computing efficiency. Art demonstrates the practical practicality of hardening learning (RL) in fine-tuned large-word model (LLM) agents for specialized high-signal use cases.
Addressing limitations in email-centric proxy workflows
Despite significant progress in retrieval augmented generation (RAG), current LLM-based agents often exhibit inefficiency when applied to structured personal data such as email. Existing methods tend to rely on general prompts and multi-tool execution, thus:
- Increased delay due to excessive processing steps
- High inference costs, especially when using proprietary models
- Variable accuracy arising from ambiguity of email content and intent
The goal behind the art is to investigate the combination of enhanced learning techniques with selected data and domain-centric designs that can improve the efficiency of agents in these dimensions.
Arts E: Architecture and Reinforcement Learning Workflow
OpenPipe has developed Art·e as a lightweight email questioning agent that integrates retrieval and generation with simplified decision-making policies. After initial supervision fine-tuning, it was trained using a proximal strategy optimization (PPO) regime and trained using an enhanced learning setup. Core components include:
- Searcher module: Identify related emails using embeddings derived from compact, efficient encoders.
- LLM Policy Leader: Generate a response informed by the search content, which is optimized by iterative RL through feedback signals.
- Evaluation pipeline: Implement automated correctness evaluation and practicality scoring to guide learning in the RL stage.
The architecture supports modularity and can independently improve or replace the hound, evaluator, or policy leader.

Evaluation: Compared with O3 agent
Benchmarking for Openai’s O3 agent on real-world email query, Artistic Proof:
Metric system | O3 Agent | Art Agent |
---|---|---|
Response Accuracy | Baseline | +12.4% |
Average delay | 1.0x | 0.2x (5x faster) |
Reasoning cost | 1.0x | 0.016x (64×cheap) |
These benefits are generated by tailored execution paths, reducing dependence on external API calls and narrower, more relevant context windows. Cost Effects tradeoffs are particularly beneficial for users to deploy agents in large-scale or privacy-sensitive environments.
Open source release and integration potential
The Art·e code base is publicly available on GitHub and provides a scalable platform for further research and practical deployment. The main functions of the repository include:
- Configurable evaluator with built-in feedback collection tool
- Abstraction of hound and language model components
- Interface to connect to a universal email provider
- Support supervised learning and through training scripts
trlx
library
This version provides a reproducible framework for applying RLHF in a proxy design in neighborhoods.
Broader meaning: RLHF in narrow proxy tasks
Although RLHF has traditionally been associated with alignment in general LLM, art embodies its applicability in narrow, goal-oriented tasks. In constrained fields such as email summary or question answering, reinforcement learning allows the agent to:
- Perform more targeted and efficient searches
- Develop a preference-perceived response policy
- Maintain robustness in noisy or partially structured data environments
Therefore, the art training approach provides a compelling path forward for organizations aimed at optimizing LLM-based agents.
in conclusion
Art·e stands for RL’s technology roots in agent development, targeting clearly defined practical problem space. Its performance improvements across precision, latency and cost metrics highlight the value of integrating enhanced learning with domain attractive system design. With growing interest in field-specific AI agents, art is a reproducible and scalable example for future R&D.
Check Github page and Technical details. Also, don’t forget to follow us twitter And join us Telegram Channel and LinkedIn GrOUP. Don’t forget to join us 90K+ ml reddit.
🔥 [Register Now] Minicon Agesic AI Virtual Conference: Free Registration + Certificate of Attendance + 4-hour Short Event (May 21, 9am-1pm) + Hands-On the Workshop
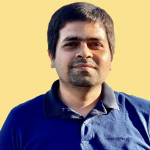
Asif Razzaq is CEO of Marktechpost Media Inc. As a visionary entrepreneur and engineer, ASIF is committed to harnessing the potential of artificial intelligence to achieve social benefits. His recent effort is to launch Marktechpost, an artificial intelligence media platform that has an in-depth coverage of machine learning and deep learning news that can sound both technically, both through technical voices and be understood by a wide audience. The platform has over 2 million views per month, demonstrating its popularity among its audience.
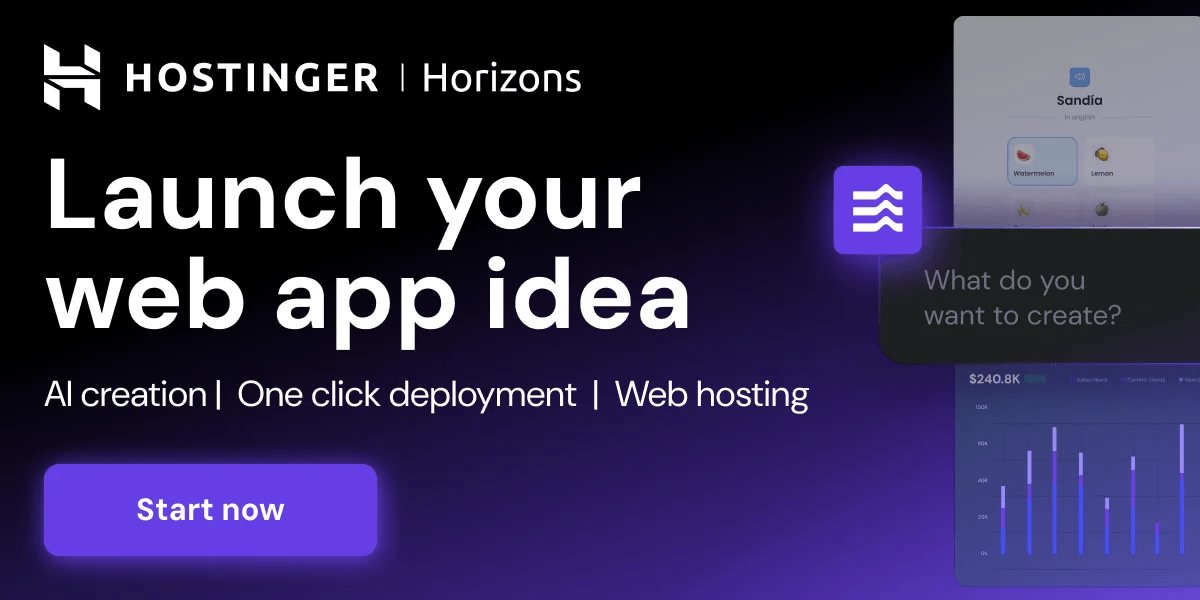