Tencent releases PrimitiveAnthing: a new AI framework that rebuilds 3D shapes using automatic regression original generation

The original abstraction of shapes breaks down complex 3D forms into simple, interpretable geometric units, which are crucial to human visual perception and are of great significance to computer vision and graphics. Although high-fidelity content can be created using latest methods of representations such as mesh, point cloud, and neural domain, they often lack the semantic depth and interpretability required for tasks such as robotic manipulation or scenario comprehension. Traditionally, original abstractions are processed using optimization-based methods that fit the shape of geometric raw materials, but are often oversegmented in semantic segmentation or learning-based methods that are trained in small category-specific datasets and therefore lack generalization. Early approaches used basic primitives such as cubes and cylinders, which later evolved into more expressive forms such as super quarters. However, a major challenge has been designing ways to abstract shapes in ways that can align different object categories while being consistent with human cognition.
Inspired by the breakthrough in the latest 3D content generation, the author proposes to redefine shape abstraction as a generation task. Their methods do not rely on geometric fitting or direct parameter regression, but instead construct the original components in turn to reflect human reasoning. This design captures semantic structure and geometric accuracy more efficiently. Previous works of automatic regression modeling (such as Meshgpt and Meshanything) have a strong effect in mesh generation by treating 3D shapes as sequences, and combine innovations such as compact tokenization and shape adjustment.
PrimitiveAnything is a framework developed by researchers at Tencent AIPD and Tsinghua University that redefines the abstraction as the original assembly generation task. It introduces a decoder-only transformer under the conditions of shape characteristics to generate sequences of variable length original sequences. The framework adopts a unified, unambiguous parameterization scheme that supports multiple primitive types while maintaining high geometric accuracy and learning efficiency. By learning directly from the shape abstraction of human-designed, the original introduction can effectively capture complex shapes that are broken down into simpler components. Its modular design supports simple integration of new primitive types, and experiments show that it produces high-quality, perceptually consistent abstractions between different 3D shapes.
The original introduction is a framework for modeling 3D mapping abstractions as sequential generation tasks. It uses discrete, unambiguous parameterization to represent each original type, translation, rotation, and scale. These are encoded and fed into a transformer, which can be extracted from the point cloud based on previous features and shape features. Cascading decoder model dependencies between properties to ensure coherence generation. Training combines cross-coagulability loss, reconstruction accuracy chamfer distances, and Gumbel-Softmax for distinguishable sampling. The process continues to conduct an automatic investigation until the end of the token signal is completed, thus allowing for flexibility and human-like decomposition of complex 3D shapes.
The researchers presented a large-scale human dataset that included 120K 3D samples with original components with manual annotation. Evaluation was performed using indicators such as chamfer distance, earth movement distance, Hausdorff distance, voxel and segmentation score (RI, VOI, SC). It shows superior performance and better alignment with human abstraction patterns compared to existing optimization and learning-based approaches. Ablation studies confirm the importance of each design component. In addition, the framework also supports 3D content generation in text or image input. It provides user-friendly editing, high modeling quality, and over 95% storage space, making it ideal for efficient and interactive 3D applications.
In short, the original introduction is a new framework that allows 3D abstractions to be approached as a sequence generation task. By learning from the original components designed by humans, the model effectively captures an intuitive decomposition pattern. It achieves high-quality results in various object categories, highlighting its strong generalization capabilities. The method also supports flexible 3D content creation using original representations. Due to its efficiency and lightweight construction, PrimitiveAntring is ideal for enabling user-generated content in games, which are essential in this application such as performance and ease of operation.
Check Paper, demos and GitHub pages. All credits for this study are to the researchers on the project. Also, please feel free to follow us twitter And don’t forget to join us 90K+ ml reddit.
Here is a brief overview of what we built in Marktechpost:
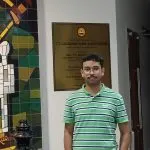
Sana Hassan, a consulting intern at Marktechpost and a dual-degree student at IIT Madras, is passionate about applying technology and AI to address real-world challenges. He is very interested in solving practical problems, and he brings a new perspective to the intersection of AI and real-life solutions.