ThinkPrm: Generation process reward model for scalable inference verification

Inference using LLM can benefit from leveraging more test calculations, depending on the high-quality process reward model (PRM) to choose promising search or ranking paths. The PRMS score problem decomposition pair indicates whether the solution is correct and has been implemented as a discriminant classifier. However, these models require a wide range of resources, including human annotations, step-by-step solutions, or computationally intensive generalization. The LLM-AS-AA-Gudge approach has advantages in data efficiency and interpretability, but they perform poorly compared to professional reward models for complex inference tasks and fail to identify incorrect inferences. This presents challenges in maintaining data efficiency and interpretability advantages while achieving superior performance of discriminatory PRM.
Research methods that address process validation challenges follow three main pathways. Discriminant PRM acts as a classifier that predicts the numerical correctness scores for each inference step, requiring extensive ladder annotation. The generated PRM framework verification is a language generation task that acts as a natural language token accompanied by verification chains (COT) to make correct decisions. These models calculate the correctness score by conditional token probability (e.g. P (“correct”)) making it inherently interpretable and scalable. Test time scaling techniques such as best N selection and tree-based search improve inference performance using additional inference time calculations. The effectiveness of these methods depends to a large extent on the validator quality of the scoring solution.
Researchers at the University of Michigan, Mira University, LG AI Research and University of Illinois Urbana-Champaign University have proposed ThinkPrm, a long-term COT validator that requires much less process labels than discriminatory PRM. It uses the inherent reasoning ability of long COT models to outperform llm-as-aaaaaaa-As-AAA-As-As-As-As-As-As-As-As-As-As-As-As-As-As-As-As-As-As-As-a-As-a-As-a-As-a-As-a-As-a-As-a-As-a-As-a-As-a-As-a-As-a-As-a-As-a-As-a-As-a-As-a-As-a-As-a-As-a-As-a-As-agudge, while using only 1% of the process labels in the PRM800K in several challenging benchmarks. Under an equally marked budget, ThinkPRM scale validation is calculated more efficiently than LLM-AS-Aa-gudge, and in the ProcessBench subset, the validation performs better than 7.2%, highlighting the value of generative, long COT PRMs for scaling test time validation with minimal supervision.
ThinkPrm is evaluated for Discprm, which uses binary cross-permeability across the entire PRM800K dataset to perform the same basic model, which contains 712K process tags from 98K problem solution pairs. Other comparisons include unweighted majority votes and validator-weighted majority for the best N experiments. The results show on three mathematical reasoning tasks: 100 questions from Mathematics 500 cover all difficulty levels, 2024 American Invitational Mathematics Examination (AIME) problems, and outdoor tasks, including physical problems for GPQA-Diamond and 200 problems for LiveCodeBench v5. For the Math-500, the researchers used the ThinkPRM-1.5B and ThinkPRM-14B together with two different generator models.
In the best N choice with the MATH500, ThinkPrm can achieve higher or comparable inference accuracy across all sampling budgets. Under a validator-guided search on the Math-500, ThinkPRM-1.5B performed about 5 percentage points better than posting and surpassed LLM-AS-AAAAAAA-Gudge using the same basic model (R1-QWEN-1.5B). Compared with Rlhfflow-Deepseek-prm and Math-Shepherd-Prm (Math-Shepherd-prm), the scaling curve of ThinkPrm-1.5b exceeded all baselines, and outperformed the performance of Rlhfflow-Deepseek-prm of 16 beams by more than 7%. For outdoor evaluation, ThinkPrm showed better scaling rates than Discprm on GPQA morphology, performing better than 8%, while on LiveCodeBench, ThinkPrm exceeded 4.5%.
In short, the researchers introduced ThinkPRM, a generative process reward model, with minimal supervised training on synthetic data, so that step-by-step verification of step-by-step reasoning can be performed. The researchers show that lightweight fine tuning of generating PRM can improve the zero-shot LLM-AS-AAA-Gudge baseline on the 8K process label. ThinkPrm also goes beyond the discriminative PRM of orders of magnitude, with more process tags, highlighting the advantages of leveraging the objectives of generating language model for interpretability, scalability, and data efficiency. The results highlight the potential of generating PRM to perform extended validation over test time, thus benefiting challenging areas such as mathematics and scientific reasoning.
Check Paper. Also, don’t forget to follow us twitter And join us Telegram Channel and LinkedIn GrOUP. Don’t forget to join us 90K+ ml reddit.
🔥 [Register Now] Minicon Agesic AI Virtual Conference: Free Registration + Certificate of Attendance + 4-hour Short Event (May 21, 9am-1pm) + Hands-On the Workshop
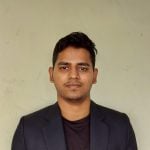
Sajjad Ansari is a final year undergraduate student from IIT Kharagpur. As a technology enthusiast, he delves into the practical application of AI, focusing on understanding AI technology and its real-world impact. He aims to express complex AI concepts in a clear and easy way.
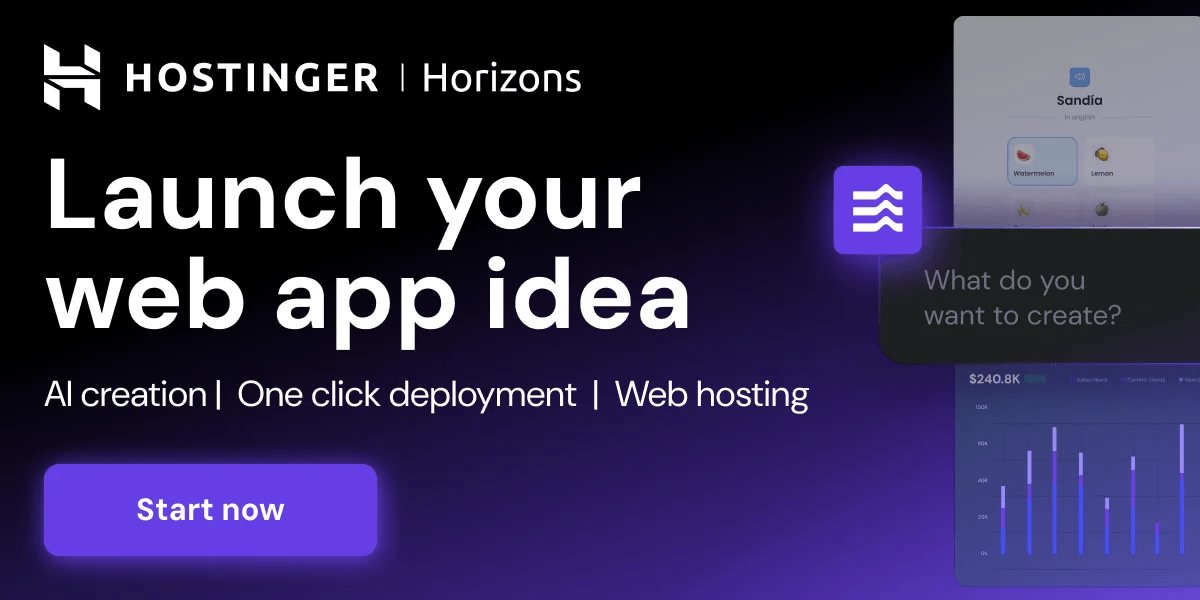