Tiny Models, Big Reasoning Benefits: USC Researchers introduce TINA to enable cost-effective enhanced learning

Despite significant progress in general task performance, achieving strong multi-step reasoning in LMS remains a significant challenge. This reasoning is crucial for complex problem-solving areas such as scientific research and strategic planning. Traditionally, enhanced inference skills involves supervised fine tuning (SFT), and models are learned by mimicking step-by-step reasoning demonstrations of more advanced models (e.g. O1). While effective, this approach depends largely on the availability of high-quality inference trajectories that are expensive and risk facilitating a shallow imitation of true logical exploration. RL provides alternatives by allowing models to learn directly from reward signals, thereby encouraging wider inference exploration. However, RL methods are often resource-rich and complex, which raises the question of how to cost-efficiently build models with inference capabilities.
After the release of powerful models, several open source work such as Stell, Sky-T1, Simplerl, Prime, and DeepScaler have explored effective strategies to replicate or exceed the inference capabilities of O1. Techniques include lightweight imitation learning, scalable instructions to tweak and simplified RL approaches. Meanwhile, new innovations such as Group Relative Policy Optimization (GRPO) improve RL training efficiency by eliminating the need for separate value networks, as shown in models such as DeepSeek-R1. To further reduce training costs, researchers are also investigating a low-level adaptability (LORA) approach, which updates only a small number of model parameters that can remain modular while maintaining inference capabilities. This method can effectively fine-tune without the calculation requirements for full parameter updates.
Researchers at the University of Southern California introduced Tina, a family of compact inference models that achieve good performance at the lowest cost. Tina enhances RL with RL on the 1.5b parameter base model to calculate the cost of a portion of the model outperforms or matches the state-of-the-art model. Their best model improves inference performance by over 20% and gets a 43.33% pass @1 on AIME24, with a cost of just $9 after training. By leveraging Lora’s efficiency to adjust the inference format while retaining basic knowledge, Tina highlights a highly accessible, cost-effective approach, with all resources fully open source.
Tina is a family of small inference models built by using the grpo-style method to use the Lora model after training in the reinforcement learning process by using the Lora model after training. The framework emphasizes minimalism-miniature models, small parameter updates, and low hardware and budget footprints. The TINA model was trained using public datasets and public datasets and replication settings for models such as Still-3, DeepScaler, and Open-R. The training utilizes the OpenR1 code base, with minimal high parameter tuning, with only two NVIDIA L40 gpus, and occasionally RTX 6000 ADA GPUs. The low cost of training and evaluation, with an average budget of $100 per experiment, makes Tina a highly accessible platform for inference research.
To ensure fair comparison, the authors reevaluated the baseline reasoning model using a consistent setup with the LightEval framework and VLLM engine, eliminating changes introduced by previous studies. Six inference benchmarks were used, including AIME 24/25, AMC 23, MATH 500, GPQA and MINERVA. They then evaluated the small, Lora-trained version of the TINA model-a small, Lora-trained version of the baseline model, showing that the Tina model usually performs better than its full-parameters than its full-participant peers despite using minimal training (19-57% of the era). Further ablation studies show that small, high-quality datasets, appropriate learning rates, moderate LORA ratings, and careful selection of RL algorithms can significantly affect performance, ensuring the efficiency and robustness of its Lora-based inference approach.
In short, Tina is a series of lightweight inference models that can leverage the least computing resources to achieve powerful performance. By applying LORA to the 1.5 B parameter base model during RL, they compete with the larger latest model, and the cost after training is only $9. The reasoning of Tina’s model is improved by 20% and the accuracy of AIME24 is 43.33%. While demonstrating impressive cost-effectiveness efficiency, there are still limitations, including smaller model scales, limited diversity in inference tasks, and minimal high-parameter adjustments. All code, logs and model checkpoints are open source to facilitate accessibility research and further exploration.
Check Paper and github pages. Also, don’t forget to follow us twitter And join us Telegram Channel and LinkedIn GrOUP. Don’t forget to join us 90K+ ml reddit.
🔥 [Register Now] Minicon Agesic AI Virtual Conference: Free Registration + Certificate of Attendance + 4-hour Short Event (May 21, 9am-1pm) + Hands-On the Workshop
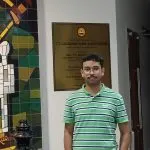
Sana Hassan, a consulting intern at Marktechpost and a dual-degree student at IIT Madras, is passionate about applying technology and AI to address real-world challenges. He is very interested in solving practical problems, and he brings a new perspective to the intersection of AI and real-life solutions.
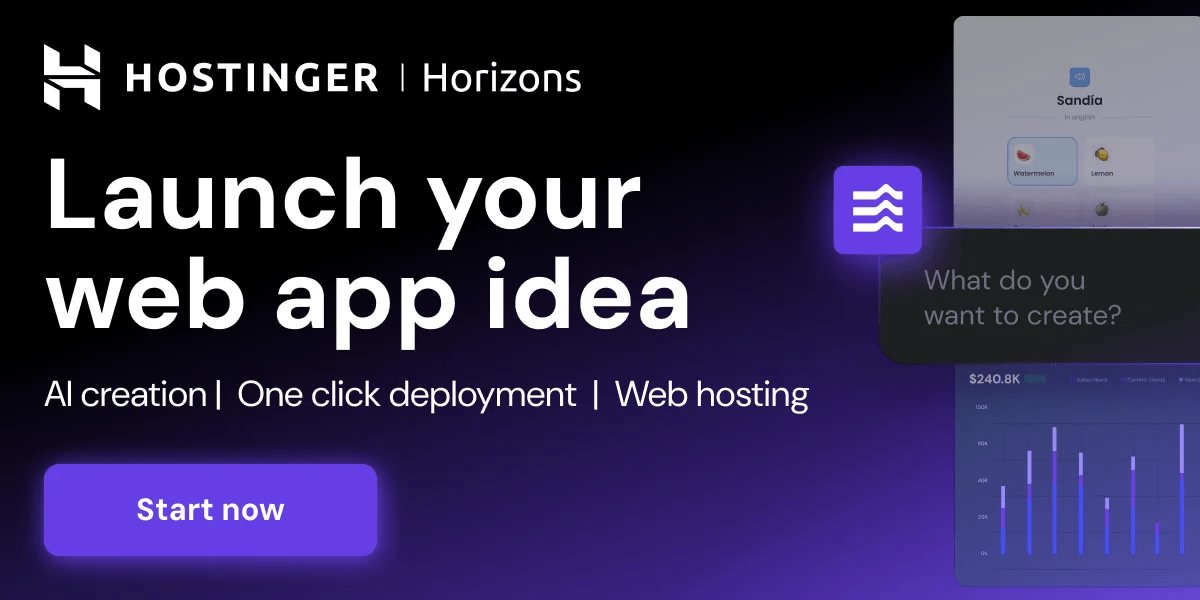