Alibaba’s QWEN team has just released the latest generation of big-word models in the Qwen3: Qwen series, offering a comprehensive bushy and hybrid expert (MOE) model

Despite significant progress in large language models (LLM), key challenges remain. Many models show limitations in subtle reasoning, multilingual ability, and computational efficiency. Typically, models are either highly capable in complex tasks, but are either slow, resource-intensive, or fast but prone to superficial output. Furthermore, scalability across languages and long post tasks remains a bottleneck, especially for applications that require flexible inference styles or long-distance memory. These issues limit the actual deployment of LLM in dynamic real-world environments.
Qwen3 just released: Targeted response to existing gaps
qwen3This is the latest version in the QWEN model family developed by Alibaba Group, designed to systematically address these limitations. Qwen3 introduces a new generation of models that are optimized for hybrid inference, multilingual understanding and effective scaling across parameter sizes.
The QWEN3 series extends the basis of earlier QWEN models, providing a wider mix of intensive combinations and expert (MOE) architectures. QWEN3 is designed for research and production use cases, with the goal of requiring applications of adaptive problems in natural language, coding, mathematics and a wider range of multimodal fields.

Technological innovation and building enhancement
Qwen3 distinguishes itself through several key technological innovations:
- Mixed reasoning ability:
Core innovation is the model’s ability to dynamically switch between “thinking” and “non-thinking” patterns. In the “thinking” mode, QWEN3 gradually conducts logical reasoning, and what is crucial is tasks such as mathematical proof, complex coding or scientific analysis. In contrast, the “non-thinking” pattern provides direct and effective answers to simple queries, optimizing latency without sacrificing correctness. - Extended multilingual coverage:
Qwen3 significantly expands its multilingual capabilities and supports more than 100 languages and dialects, thereby improving accessibility and accuracy in various locales. - Flexible models and architectures:
The QWEN3 series includes models with 500 million parameters (density) to 235 billion parameters (MOE). Flagship model, QWEN3-235B-A22Bactivates only 22 billion parameters per reasoning, which can provide high performance while maintaining manageable computational costs. - Long context support:
Some QWEN3 models support context windows 128,000 tokensenhance their ability to handle lengthy documents, code bases and multi-transfer conversations without degrading performance. - Advanced training dataset:
QWEN3 utilizes a refreshed, diverse corpus with improved data quality control designed to minimize hallucinations and enhance cross-domain generalizations.
Additionally, the QWEN3 basic model is released under an open license (constrained by specified use cases), enabling research and open source communities to experiment and build.
Experience results and benchmark insights
The benchmark results show that the QWEN3 model is competitive with its leading contemporaries:
- this QWEN3-235B-A22B The model achieves strong results in coding (HumaneVal, MBPP), mathematical reasoning (GSM8K, math) and common sense benchmarks, with DeepSeek-R1 and Gemini 2.5 Pro series models.
- this Qwen3-72b and qwen3-72b-chat The model demonstrates reliable follow-up and chat capabilities, showing significant improvements with the early QWEN1.5 and QWEN2 series.
- It is worth noting that QWEN3-30B-A3BThis is a smaller MOE variant with 3 billion active parameters, which outperforms QWEN2-32B on multiple standard benchmarks, indicating increased efficiency without an accurate trade-off.

Early evaluation also showed that the QWEN3 model exhibited lower hallucination rates and more consistent multi-steering dialogue performance compared to previous Qwen generations.
in conclusion
Qwen3 represents a thoughtful evolution in the development of large language model. By integrating hybrid inference, scalable architecture, multilingual robustness and effective computing strategies, QWEN3 solves many core challenges that continue to impact today’s LLM deployments. Its design emphasizes adaptability – it also applies to academic research, enterprise solutions and future multimodal applications.
Instead of providing incremental improvements, QWEN3 redefines several important aspects of LLM design, setting new reference points for balancing performance, efficiency, and flexibility in increasingly complex AI systems.
Check Blog, model embracing faces and github pages. Also, don’t forget to follow us twitter And join us Telegram Channel and LinkedIn GrOUP. Don’t forget to join us 90K+ ml reddit.
🔥 [Register Now] Minicon Agesic AI Virtual Conference: Free Registration + Certificate of Attendance + 4-hour Short Event (May 21, 9am-1pm) + Hands-On the Workshop
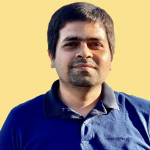
Asif Razzaq is CEO of Marktechpost Media Inc. As a visionary entrepreneur and engineer, ASIF is committed to harnessing the potential of artificial intelligence to achieve social benefits. His recent effort is to launch Marktechpost, an artificial intelligence media platform that has an in-depth coverage of machine learning and deep learning news that can sound both technically, both through technical voices and be understood by a wide audience. The platform has over 2 million views per month, demonstrating its popularity among its audience.
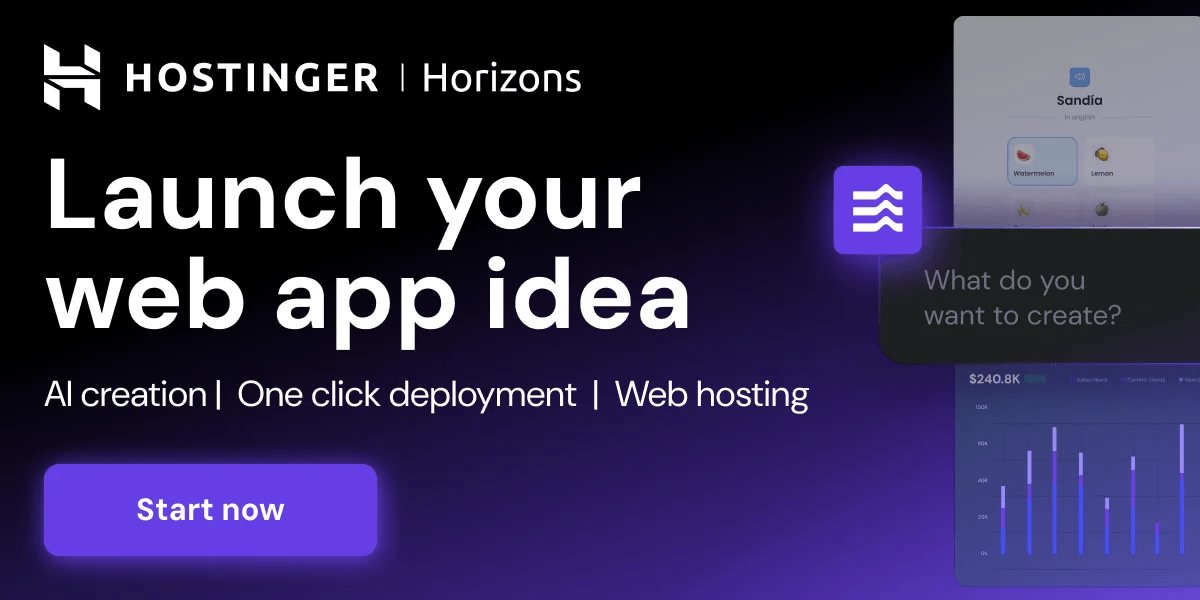