Wild introduces SEED1.5-VL: a basic model of visual language aimed at improving universal multimodal understanding and reasoning

VLM has become the core of building universal AI systems that can understand and interact in digital and real world. By integrating visual and text data, VLM has made progress in multimodal reasoning, image editing, GUI proxying, robotics and more, thus affecting departments such as education and health care. Despite this advancement, VLM still lags behind human abilities, especially in tasks involving 3D inference, object counting, creative visual interpretation, and interactive gameplay. Unlike the rich text resources available to LLM, the challenge lies in the scarcity of scarce, diverse multimodal datasets. In addition, multimodal data complexity presents significant training and assessment barriers.
Researchers at BONDENACE have developed Seed1.5-VL, a compact and powerful visual foundation model with a 532 M parameter visual encoder and 20 B parameter Experts LLM. Despite its efficient architecture, SEED1.5-VL achieved the highest results in 38 of 60 public VLM benchmarks, performing well in tasks such as GUI control, video comprehension, and visual reasoning. It was trained on trillions of model tokens using advanced data synthesis and post-training technologies, including human feedback. Innovations in training (such as hybrid parallelism and visual token redistribution) optimize performance. The model’s efficiency and powerful inference capabilities are suitable for interactive applications in the real world, such as chatbots.
The SEED1.5-VL architecture has a visual encoder, an MLP adapter and LLM. Its custom visual encoder seed weight, supports local resolution image input using 2D ropes and imaged through 14×14 patches, followed by average pooling and MLP. Preprocessing involves masked image modeling, contrast learning, and Omni pattern alignment using image, text, and video fit pairs. The model uses a dynamic framework resolution sampling method for video encoding to adapt frame rate and resolution based on content complexity, balancing efficiency, and detail. This approach allows for an efficient space-time understanding within the token budget, ensuring a comprehensive video representation across different lengths and complexities.
Pre-training of seed 1.5-VL involves curating 3 trillion high-quality tokens in different fields. Image text pairs were performed from the network using clip scores, size/aspect ratio checks and deduplication to reduce noise. Using domain-based sampling and repetition strategies, rare visual concepts solve class imbalances too much. Use images, charts and tables with annotated and synthetic texts to add a dedicated dataset for OCR – Object grounding and counting tasks to use bounding boxes, points and automatically labeled web data. Other tasks include 3D spatial understanding using deep annotations, and video understanding that supports dynamic content analysis through multi-frame subtitles, QA and temporal grounding.
This evaluation highlights the competitive performance of seed weight and seed 1.5-VL in visual tasks. Although seed weapons have significantly fewer parameters, they may be better than larger models such as intervl-c and eva-clip, showing high accuracy and robustness on datasets such as ImagEnet-A and ObjectNet. SEED1.5-VL shows powerful capabilities in multimodal reasoning, general VQA, document comprehension and grounding. It implements the latest benchmarks, especially in complex inference, counting and graph interpretation tasks. The model’s “thinking” pattern combines longer chains of reasoning, further improving performance, showing its powerful capabilities in detailed visual understanding and task generalization.
In short, Seed1.5-VL is a visual basic model with a 532 M parameter visual encoder and a 20 B parameter Experts language model. Despite its compact size, it still gets the latest results in 38 of 60 public benchmarks and excels in complex inference, OCR, graphical interpretation, 3D spatial understanding and video analysis. It also performs well in proxy-driven tasks such as GUI control and gameplay, surpassing models like Openai Cua and Claude 3.7. The model shows a strong generalization of tasks beyond its training range. The study outlines its architecture, data pipelines, and training approaches and identifies future directions, including enhanced tool usage and visual reasoning capabilities.
Check Paper and project pages. All credits for this study are to the researchers on the project. Also, please feel free to follow us twitter And don’t forget to join us 90K+ ml reddit.
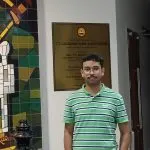
Sana Hassan, a consulting intern at Marktechpost and a dual-degree student at IIT Madras, is passionate about applying technology and AI to address real-world challenges. He is very interested in solving practical problems, and he brings a new perspective to the intersection of AI and real-life solutions.
